Over the past years, the quant industry has changed substantially. My first visit to Global Derivatives was just over a decade ago. At the time, perhaps unsurprisingly, the conference was dominated by presentations about option pricing. Over the years, the content has become more varied, and indeed the name was changed to QuantMinds a few years ago. Whilst the traditional content, involving option pricing is a large part of the event, other topics have become more significant, notably machine learning, which has percolated through both the sell side and buy side. In general, the attendence has become a bit more mixed, with more buy side attendees compared to a decade ago. QuantMinds is also now regularly hosted alongside RiskMinds. This year the combined event returned to London. I presented at the event, and also many of the talks. There were many talks and roundtables at the event, far too many to feature in this relatively short article, but I’ll nevertheless try to write about a few of my takeaways from the event, which I hope offers a very snapshot of the event. (Before anyone asks, whilst, there is no further mention of burgers within the article, I do note that they were served at the buffet!)
A few takeaways from QuantMinds, starting with generative AI and LLMs
Generative AI and LLMs have been everywhere over the past few years. Finance as a field has looked at ways of incorporating LLMs. Theo Lau (Unconventional Ventures) chaired a panel on the subject with Gary Kazantsev (Bloomberg), Dan Nechita (formally at the European Parliament), Rama Cont (Oxford University), Nicole Koenigstein (quantmate) and Stefano Pasquale (BlackRock). Nechita began the panel by discussing the impending regulations for AI which were coming into force in the near term in EU, and contrasting them to the executive order signed in the USA which regulates AI based on government contracting. Konigstein said that there were now many use cases in production, whether it was improving cybersecurity, in corporate lending, where it enabled you to go through thousands of documents in seconds etc.
Whilst there is a massive buzz around the topic, Kazantsev suggested more broadly there was an overemphasis on LLMs. They were for example not the best models for time series. Whilst standard examples could work with LLM, the performance can degrade, if the topic isn’t involving memorization. Indeed, they do not compute closures. If you need to guarantees and proves for your output, you need to build something more complicated. In time series forecasting, interpretability is required to prove explanation, and we can’t real use a giant transformer for that. Cont continued along the theme that there was too much emphasis on LLMs. Generative AI proceeded before LLMs. There was huge potential for generative AI (ie. non parametric simulation algos), given that simulative was everywhere in risk. He also noted that a lot of the publications using these models in finance, were using irrelevant benchmarks. Quants want reliability and accuracy. Pasquele chimed in noting that most of the papers from his team was focused towards interpretability.
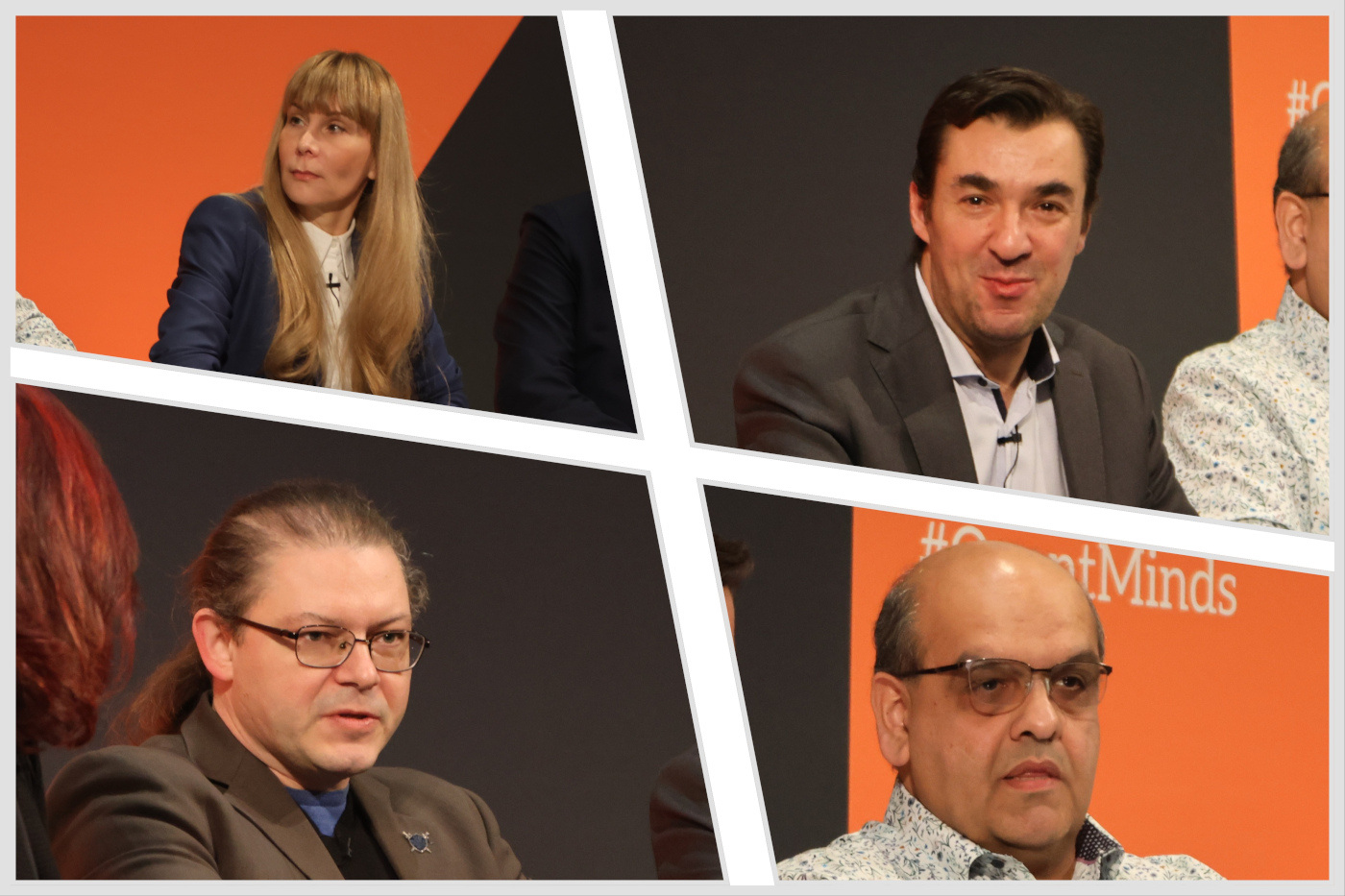
Nicole Konigstein (quantmate), Dan Nechita (former European Parliament), Rama Cont (Oxford University) and Gary Kazantsev (Bloomberg) – clockwise
Alexander Sokol (Compatibl) continued the LLM theme in his presentation, given a particular use case for them, namely to extract trade entry data from unstructured messages such as e-mails. Indeed, this is something that is done daily across trading floors worldwide everyday. It is a necessity to do. The outcome of the exercise is an open source library tradeentry (on GitHub). Traditionally, this problem of trade entry is done manually, but up until now it hasn’t really been approached from the angle of LLMs, and hasn’t really been a priority for foundation model developers. This contrasts to code generation in languages such as Python, which has seen the advent of tools like GitHub Copilot. Furthermore, it requires domain specific knowledge from the capital markets. One thing he noted was that LLMs behaved and performed like humans. Perhaps therefore it wasn’t surprising, that like human they could make mistakes, and like humans they needed “mentoring” to fix issues. He suggested the best approach is to use a divide and conquer approach to increase the reliability of the outcome, in particular, to avoid scenarios such as an LLM hallucinating something like a notional, or to guess fields which were not originally specified in the trade message.
Reviewing the year
Eric Vynckier (Foresters Friendly Society) moderated a panel with Anton Memushkin (Jain Global), Lucette Yvernault (Fidelity International) and Aitor Muguruza Gonzalaz (Kaiju Capital Management), which reviewed the past year from a markets, quant and technology perspective. Gonzalez noted that AI stocks had been driving the market, but we were moving away from that paradigm. It had been a more interesting environment for mean reverting strategies, and vol was still low, although he did note that VIX doesn’t stay at 10% type levels for too long. More broadly, we were back to a more attractive environment for systematic strategies. Other markets such as India had also become more attractive, given they were experiencing a technological revolution. Yvernault discussed fixed income and credit markets, noting that investment grade had been good. The interest rate component had been a volatile component. Dispersion in the various names had been good. On the data side, Memushkin suggested that market data was very important when modelling markets, risk factors etc. but so was the experience of people.
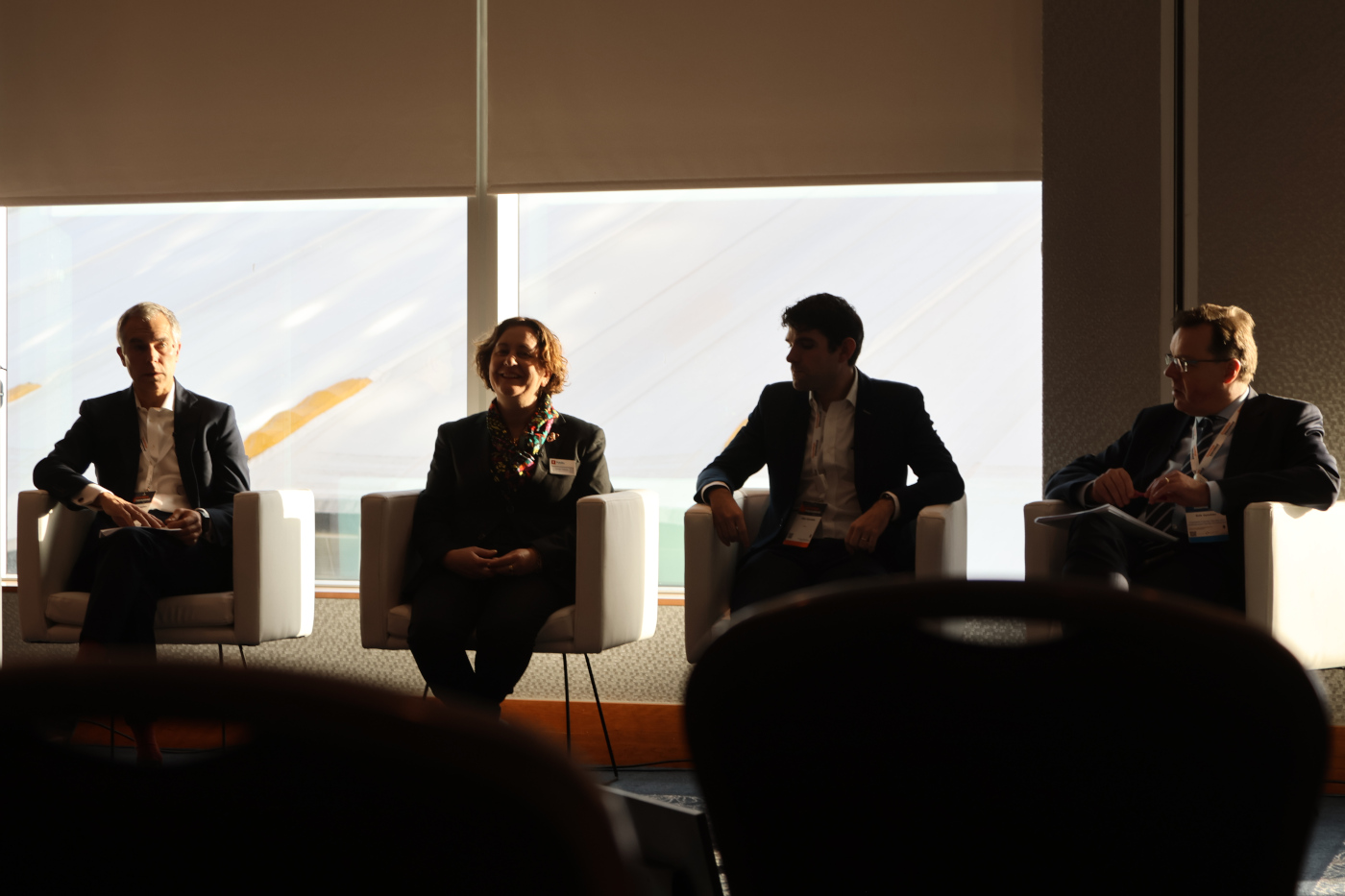
Anton Merlushkin (Jain Global), Lucette Yvernault, (Fidelity International), Aitor Muguruza Gonzalez & Erik Vynckier (Foresters Friendly Society) – left to right
Dealing with nonstationary data and ideas for time series
In his presentation, Paul Bilokon (Thalesians and Imperial College) discussed the optimal allocation of resources in nonstationary conditions. Indeed, nonstationarity is one of the problems which makes any financial markets “difficult”. Relationships between assets are not “stationary” and correlations are themselves volatility, and the market has different regimes. He outlined how Markowitz was well aware of the issues associated with his method of optimising portfolios, in particular, it is difficult estimate Sharpe ratios. He suggested solutions such as optimal allocation with Sharpe ratios in the context of reinforcement learning and bandits. He also outlined joint research with his students, looking for techniques to understand regime classification, with a particular application looking at trading rules for iTraxx CDS indices.
I also gave a talk about forecasting economic time series in particular inflation, using machine learning, which is something we do at Turnleaf Analytics. I noted how using larger datasets could improve the forecasts from more traditional approaches, and in particular augmenting our datasets with “alternative data” to give us a richer insight into how the economy was performing. I later presented several trading strategies for DMFX, EMFX and commodities, which used our economic forecast data as an input. I showed, how they had been largely profitable out-of-sample/after publication. Indeed, I think it’s a very important point when publishing research, to track model performance after publication, and it is something I’m doing much more regularly, when I have access to data on an ongoing basis.
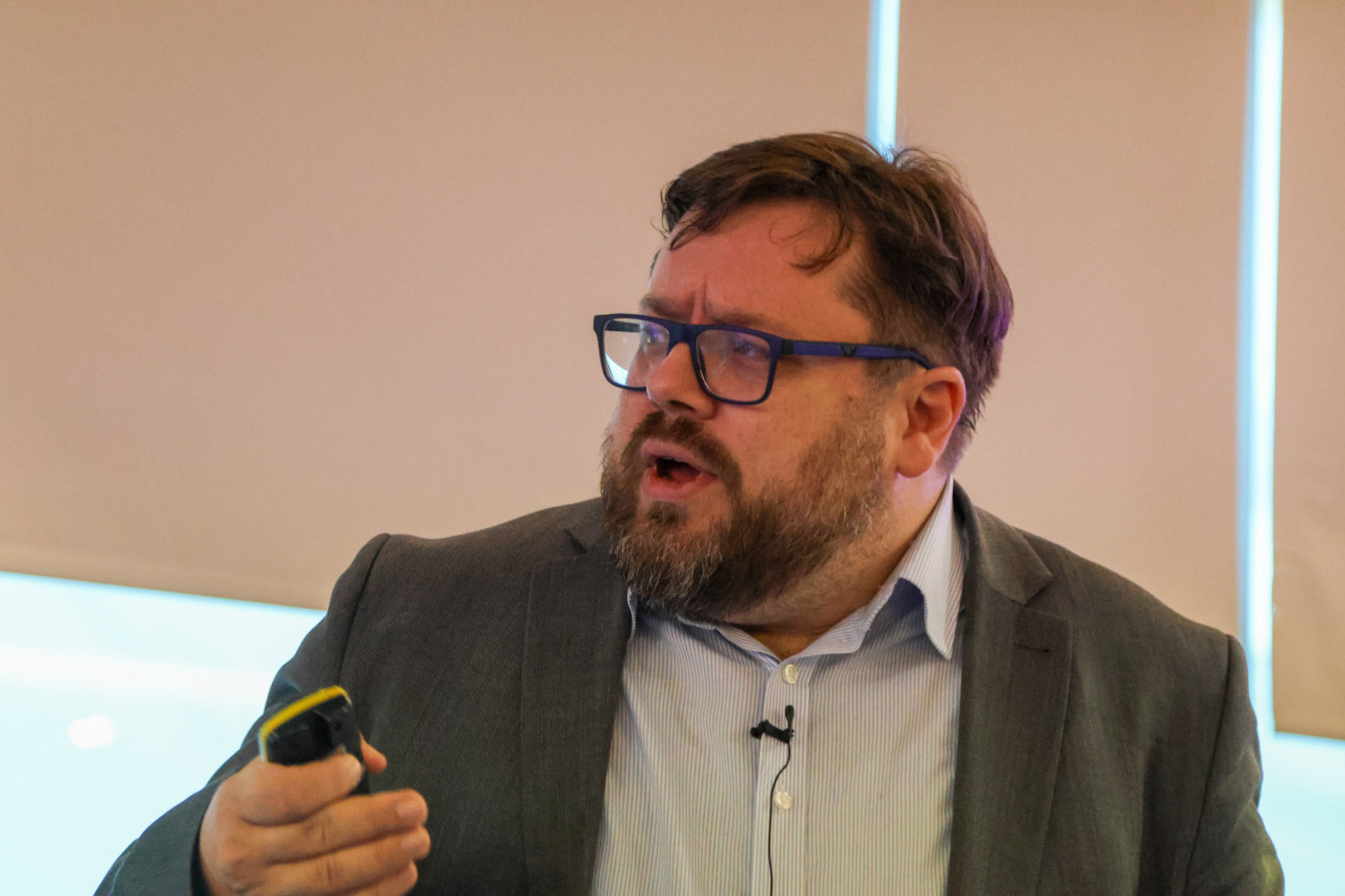
Paul Bilokon (Thalesians and Imperial College)
The commodities angle, bitcoin and climate change
Helyette Geman (John Hopkins) focus was on commodity markets in particular crude oil and and also the market for fertilizers. She noted how energy had changed over recent centuries, with wood giving way to coal, which itself was being displaced by oil and latterly natural gas, for the generation of electricity. More recently renewables had come to the fore. LNG had become more important and was being actively traded. Elsewhere she discussed how fertilizers could be traded using trading rules associated with equities which were heavily linked to fertilizers. More broadly, she noted how substitution in commodities could lead to correlations such as with wheat and corn.
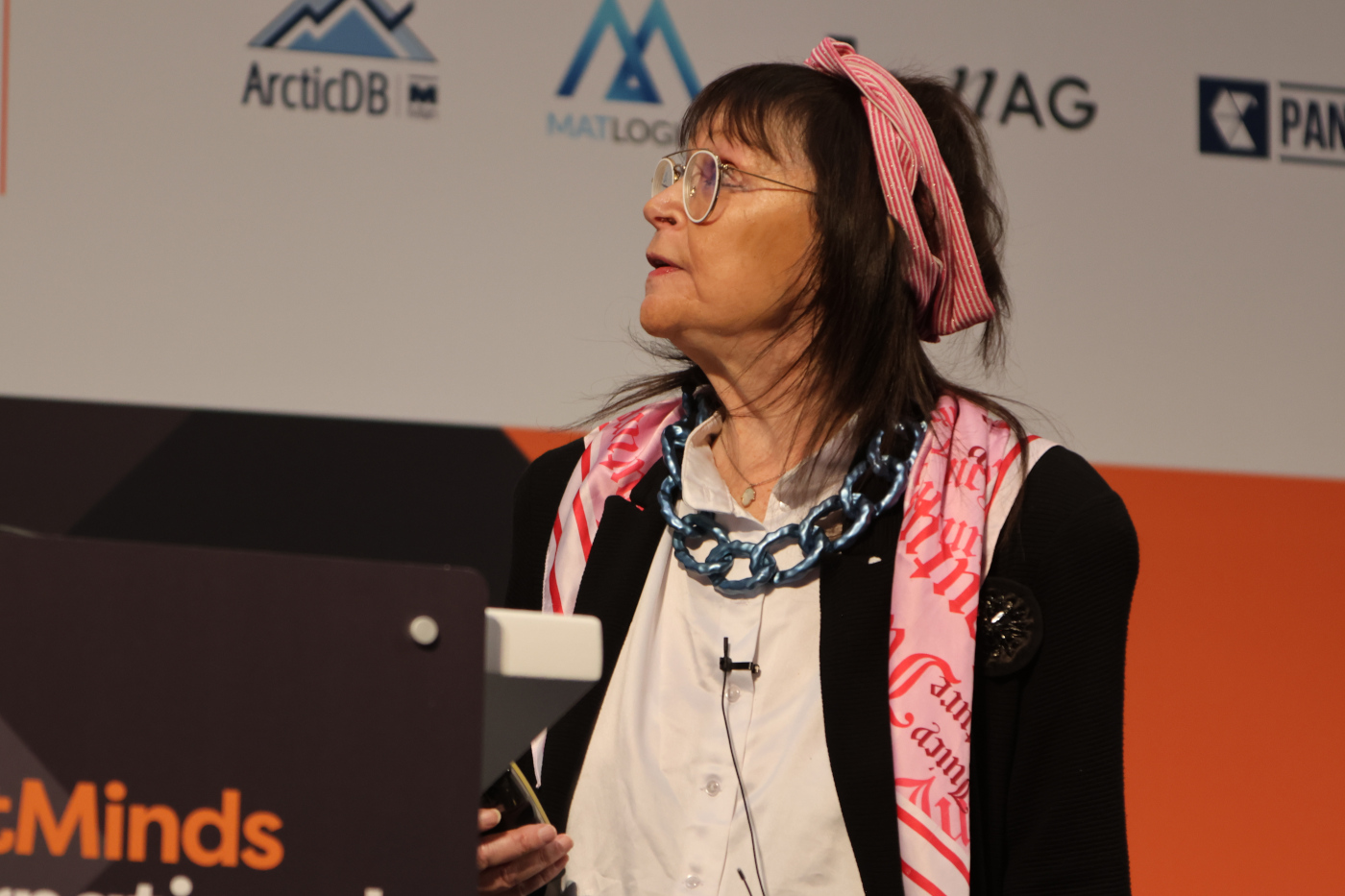
Helyette Geman (John Hopkins)
With bitcoin knocking on the door of 100k USD this week, Carol Alexander (Sussex University) gave a very topical talk on the order flow impact and price formation in centralized crypto exchanges. Her study looked at Binance and Coinbase order book data (L2 and L3 respectively), with timestamps with millisecond precision. She noted that bitcoin did not have fundamental value, and was a speculative asset, although she noted there were differing opinions on this point. Ethererum by contrast had some value associated with its utility associated with smart contracts. My own personal view is that its ultimately a speculative asset. If there’s enough people who believe it has value, it will inevitably have value, even if there can be some specific use case. In a sense, it is like gold in that aspect. Yes, gold does have some utility, such as for electrical cabling or jewellery, but it’s utility as a storage of value/investment vehicle outweighs that. Gold does of course have somewhat of a head start in historical terms though! Her paper suggested that orders on the same side as the imbalance have greater impact. Market integration tended to break down at very high frequencies, although, I wonder how much of that could be attributing to factors like bid/ask bounce? There were differences in the microstructure of the exchanges owing to different spreads, costs for market makers etc. For example, price changing actions tended to be less frequency on Binance because spreads were tighter there.
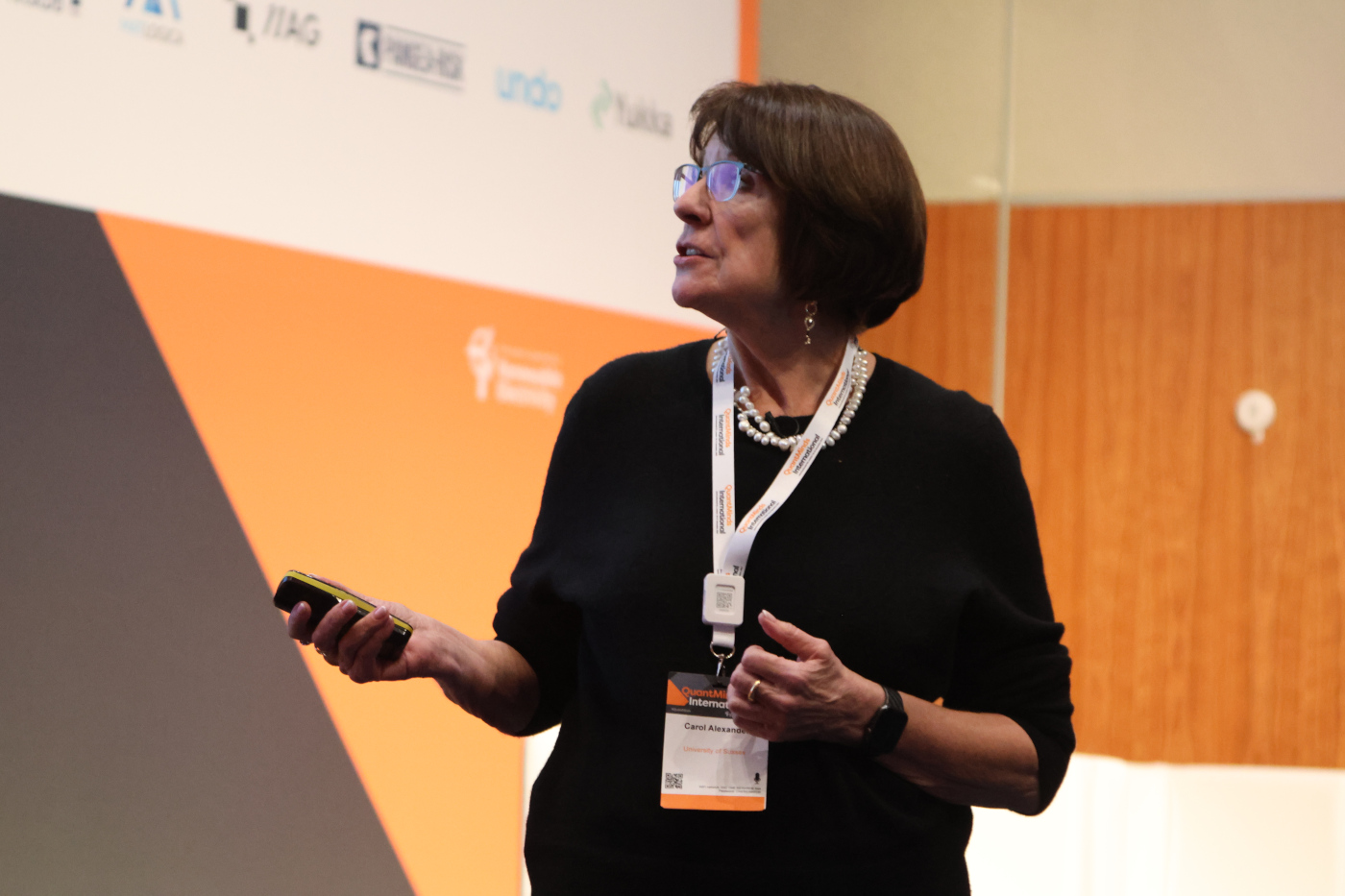
Carol Alexander (Sussex University)
If gen AI is one buzzword, so is ESG! There was a panel on the topic of breaking down sustainability for quant finance, moderated by Richard Turner (Mesirow), and with panellists Andrea Macrina (UCL), Edward Baker (Tipping Frontier) and Ying Poikonen (SMBC). Given the recent backlash towards the topic, it was noted how investment managers would adjust their pitches to their audience (pitch books with and without ESG). More broadly, green washing was an issue. Whilst the problem with the long term challenge in climate change, it needed short term decisions. One way to incentivise the “E” in ESG, was by offering incentives and on the other hand penalties, it was suggested. There were many challenges when it came to modelling supply chains, which was important when trying to understand the impact of climate change on a portfolio. In many cases, investment managers don’t often known the physical location of suppliers in a supply chain.
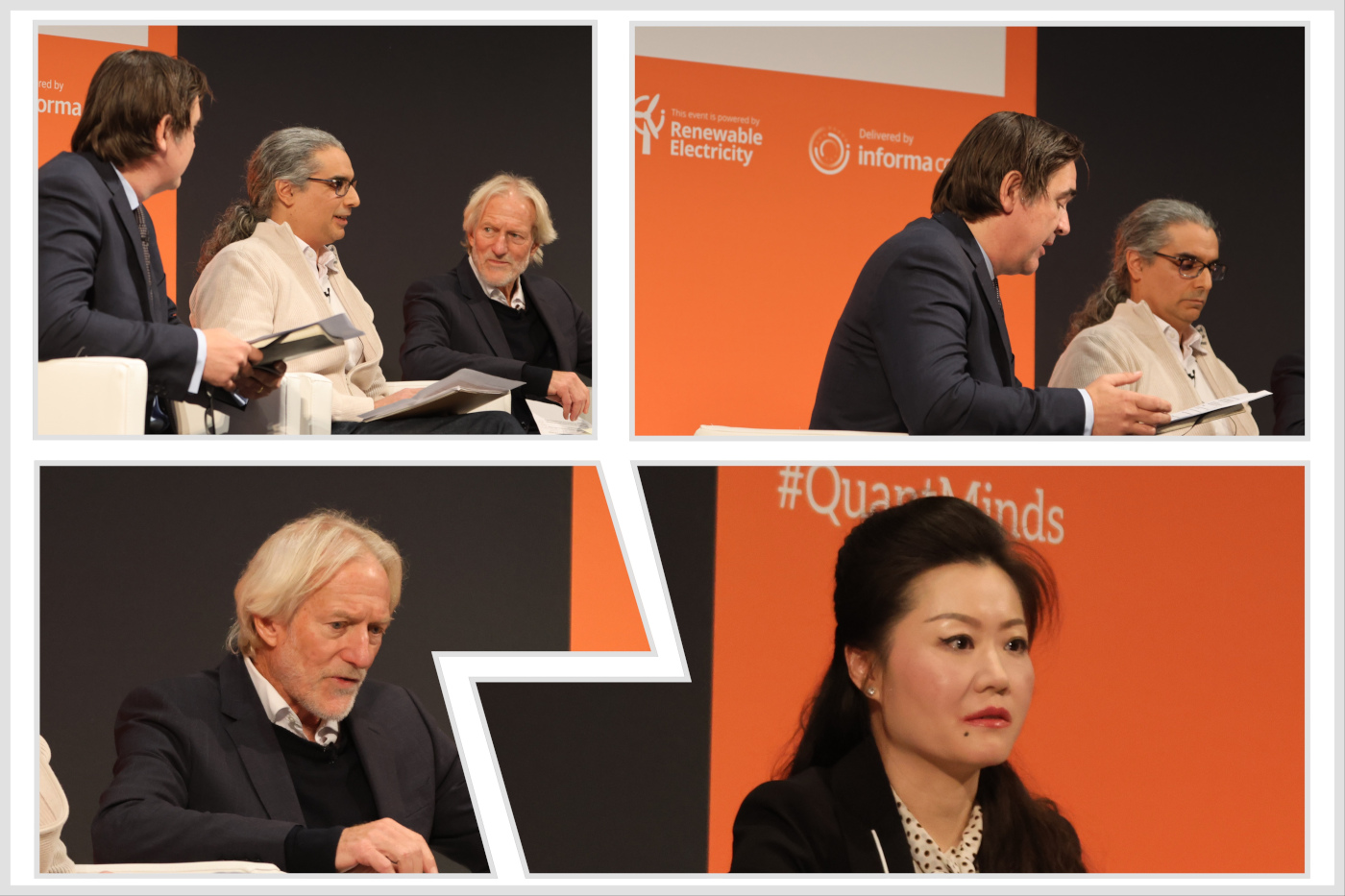
Andrea Macrina (top left in the middle – UCL), Richard Turner (top right – Mesirow), Edward Baker (bottom left – Tipping Frontier) and Ying Poikonen (bottom right – SMBC)
Women in quant
The Women in Quant panel was chaired by Samar Gad (Kingston Business School), with panellists Laura Lise (Citi), Elissa Ibrahim (EBRD) and Svetlana Borovkova (Bloomberg). It’s hardly a surprise to anyone who has worked on a trading floor that female quants are a much smaller proportion of the workforce compared to male quants, indeed one figure quoted in the panel suggested that only around 20% of quants are women in the UK. One point of discussion was that this was at odds with the fact that 40% of STEM graduates are female. It was suggested that this divergence started much earlier at school. Indeed in tech, women are more prominent than they are in quant, it was noted. One way to help was to offer mentorship at early stages, and initiatives like the Women in Quant Finance group on LinkedIn started by Wafa Schieffer helped.
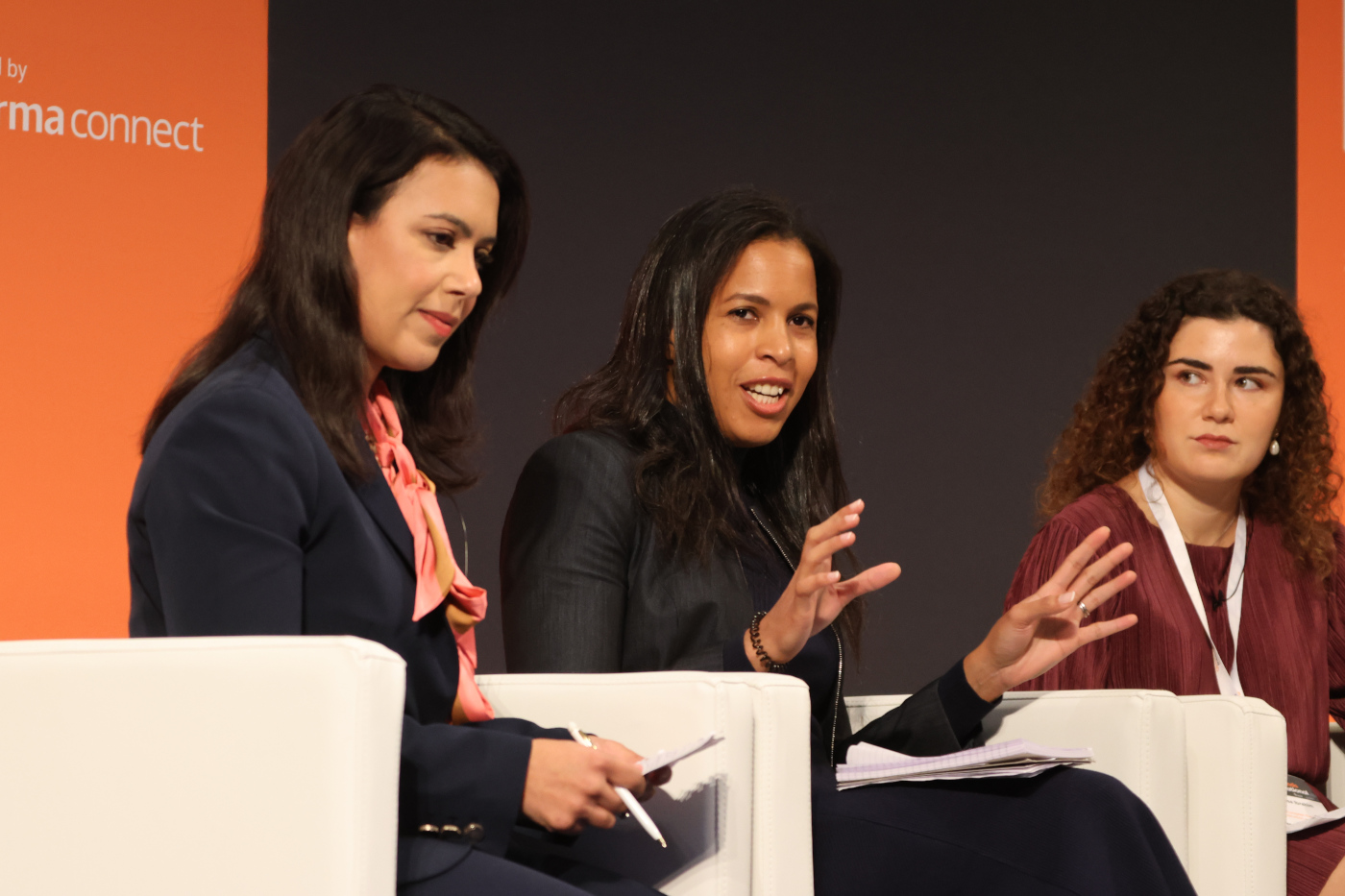
Samar Gad (Kingston Business School), Laura Lise (Citi) and Elissa Ibrahim (EBRD)
Dealing with data in finance and beyond
James Munro (Man Group) talk centred around hard learned advice on scaling data for quants. He noted that quant is all about data science. It was important to understand and optimise you data management process and how people fit into it. It was valuable to catalogue datasets that a firm had been using and also those datasets that had been discarded (and reasons why). Teams using data could be split in many ways, and often they could straddle a number of different tasks. He stressed that when it came to data, there is no substitute for figuring it yourself. he compared and contrasted using tools like SQL for storing time series versus solutions dedicated to storing them such as Man Group’s own ArcticDB, which did the computation more efficiently for such data structures.
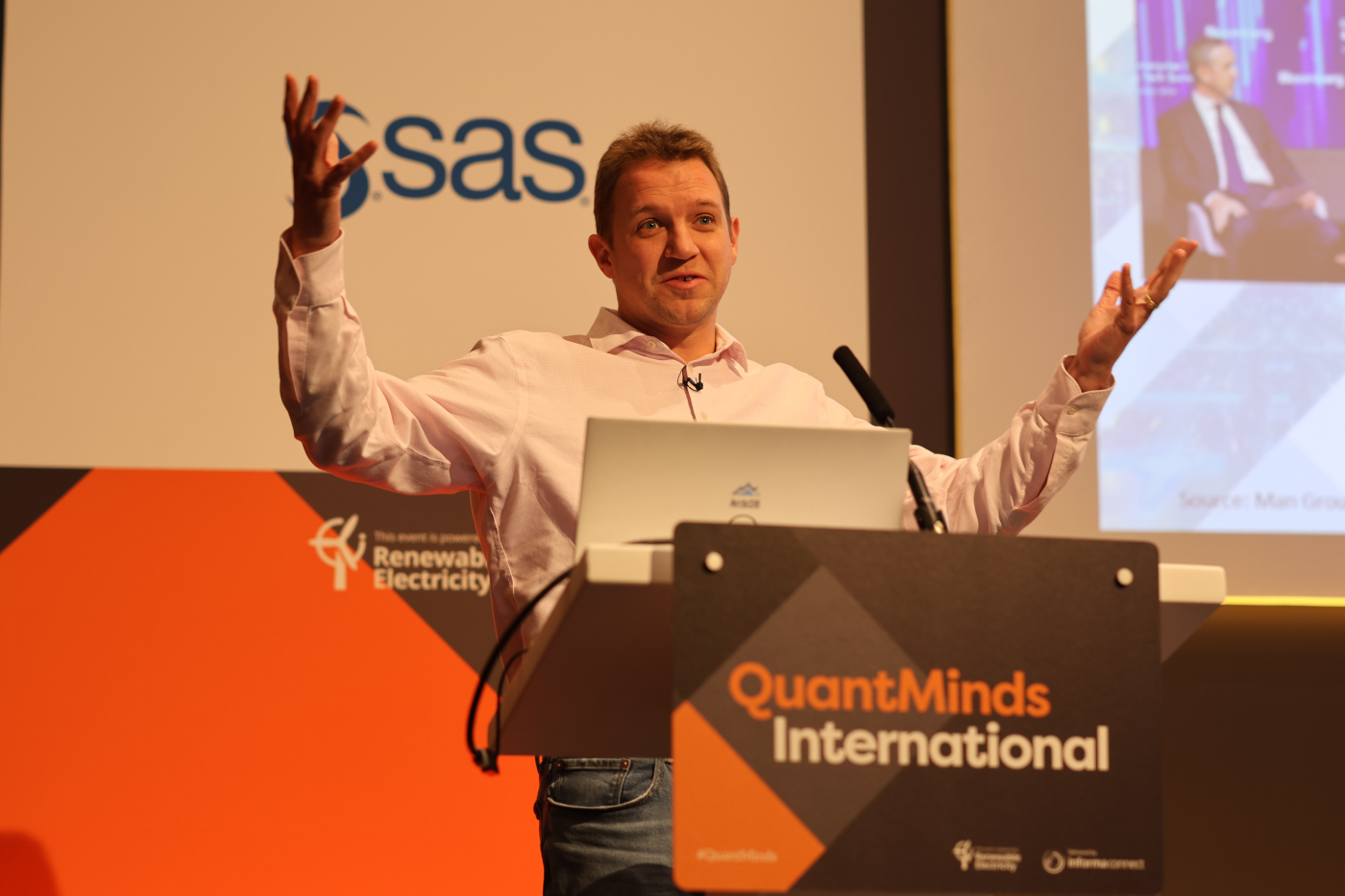
James Munro (Man Group)
In finance, we often deal with large datasets. They require a lot of storage capacity and need large computation requirements. However, it is all a matter of perspective. For high frequency traders, the mountain of data is huge compared to that used by folks utilising daily data for example. However, I would conjecture that in general, the amount of data we deal with, is far less than that generated at CERN. Joachim Mnich (CERN) gave a talk outlining the challenges with dealing with the data from CERN’s particle accelerator. At present they generate over 100 PB a year. They store around 1500 PB of data worldwide. They have the worldwide LHG Computing Grid, consisting of a million computational cores in around 160 data centres worldwide. They were actively using deep learning and AI in their computation. They had very long time horizons when it came to creating processes to crunch the data, and the amount of data being computed was going to be much bigger when new colliders are built (planned to be 91km long versus the current 27km collider).
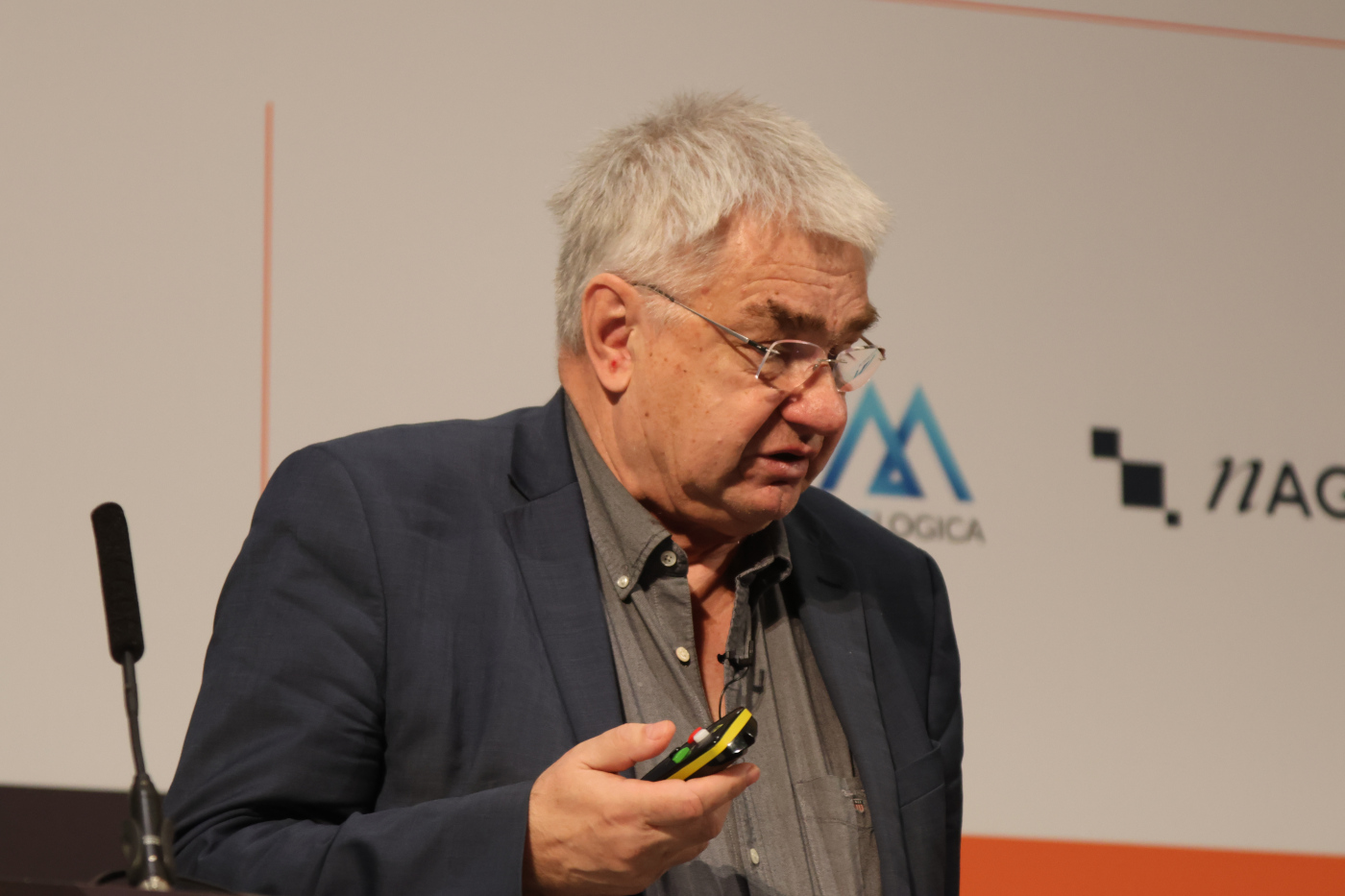
Joachim Mnich (CERN)
ConclusionQuantMinds has been a great event over the past week. It’s definitely obviously where quant is going from the various presentations and discussions at the event. Whilst a few years ago machine learning was very much in the “research” phase, it’s now clear that it is being used actively across many different areas of the financial industry by quants. Tools like LLMs do give rise to a lot of possibilities. However, at the same time, the way they behave does make them more challenging to use in a systematic setting (ie. the hallucinations and the lack of consistency in their output), although we did see some approaches to alleviate this issue in some of the sessions at QuantMinds. It will be interesting to see how QuantMinds evolves in the coming years. I do however suspect that LLMs might not be such a hot topic in the years ahead. Anyway, let’s bookmark that thought, and come back to it in a few years!