When you’re in New York, there are several things you have to have, a burger (preferably from Minetta Tavern), a cookie (preferably from Levain bakery on the Upper East Side) and a bagel (preferably from my new favourite, Popup Bagels). However, I promise this article is not an exercise in culinary product placement. As well as traversing my way through New York from bagel to cookie, I recently attended the Neudata summer conference in New York, several weeks after the London edition.
I had last attended Neudata New York many years ago, so I was keen to see how the event has evolved. Neudata’s conferences have become some of the most important events on the calendar, when it comes to finding “new data” (pun totally intended) for financial markets, and in particular alternative data. That being said, the company also has an event purely focused on traditional data these days. Whilst, the London and New York events do bear similarities, and are substantially larger than they were in years gone by, the attendance is quite different. Perhaps unsurprisingly, the New York event tends to get skewed more towards US based folks and the UK event is very much more filled with European attendees. As a result, the New York presentations, panels and general discussions during the breaks, ended up being quite different to ones in London.
In this article, I’ve tried to put together some of my takeaways from a small number of various presentations and panels I attended at Neudata New York. For brevity I haven’t gone through every single session.
Kicking off the event, with a recap of alt data today
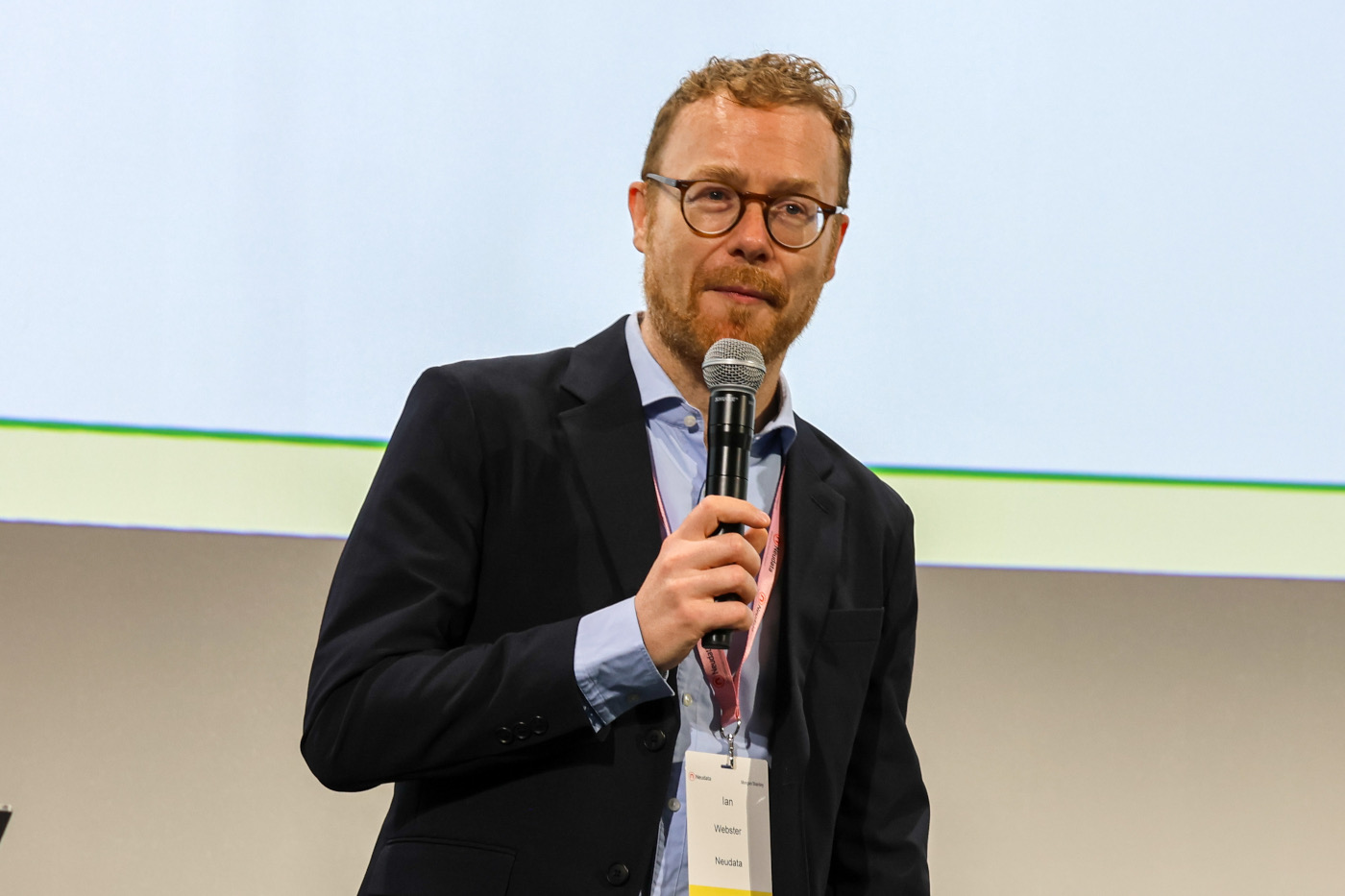
Ian Webster, Neudata
Ian Webster, Neudata, kicked off the event discussing some trends in the data space in 2025. Tariff talk has unsurprisingly impacted data markets, with folks looking for datasets such as bills of lading data. More broadly there had been data vendor consolidation, such as Consumer Edge’s acquisition of Earnest Analytics. Amongst the various data vendors there has also been a drive in terms of product development, notably to produce higher frequency datasets. We’ve definitely seen this at Turnleaf Analytics, where there we’ve increased the frequency for our inflation forecasts to weekly for the major economies and daily for on of our US inflation forecasting models. Webster also highlighted some new interesting datasets for GPU cloud pricing and also South African consumer transaction data.
Keynote interview: Mark Fleming-Williams interviewing Abraham Thomas
Anyone who has been involved in financial data over the past few years will have heard of the data company, Quandl, which was acquired by Nasdaq in 2018. In this session, Mark Fleming-Williams, CFM, and also host of the Alternative Data Podcast (a must listen for anyone in this space) interviewed Abraham Thomas, co-founder of Quandl (along with Tammer Kamel), and as Fleming-Williams described him the “alt-data godfather”. Abraham started by describing his career on the buy side first in Tokyo and then in New York as a PM. Whilst he wanted to make data driven decisions, it was hard to get the data. He wanted to do something about it, and hence coded up a website that could host any dataset with a simple API to use, where you could buy individual datasets. I’ve personally used Quandl a lot in the past, and you could find a myriad of both market data and alternative data, all with a nice easy to use API. It was one of the first data marketplaces in our industry. Since exiting Nasdaq Data Link (as Quandl was renamed) in 2021, Abraham has been an angel investor and also active in publishing his thoughts on the data industry on Substack (which I strongly recommend you subscribe to).
Impact of LLMs
Fleming-Williams then went on to asking Abraham how alt data had changed over the years. Abraham noted that alt data was a category in its own right, and we were now beyond the education phase of having to describe precisely what it was. However, the space was still dominated by hedge funds, as it had been before. Unsurprisingly, LLMs has impacted data space. Abraham stated that the value accrues to whoever controls the scarce resource. Before LLMs, software was scarce. Instead of it was data that was abundant. We all leave a trail of data, with whatever activity we do online or purchase, whether it’s credit card data etc. Given the cost of storage is so low, it’s been possible to store everything. Abraham suggested that ultimately software and data are two sides of the same coin. However, given that LLMs are so hungry for data, we now have data scarcity.
LLMs commoditise a lot of work, Abraham continues. What was once quite cumbersome to do can be automated, but of course that’s true for everyone else. Having data that only you have access to and having IP which LLMs cannot replicated, was key. LLMs can also open up brand new avenues which were not possible before.
The theme of LLMs continued later in a talk by Qingyi Huang, Morgan Stanley. Huang described the use of LLMs to extract insights in earning calls. The idea was to use LLMs to convert paragraphs into text embeddings (ie. into a numerical form), and use that to do analysis. By use the embeddings directly, results were more reproducible.
New vendor showcase and the impact of Trump on the industrial sector
As ever, one of the main parts of a Neudata event is a collection of presentations to showcase new data vendors. One which I found particularly interesting was CredolQ, which collected data from TikTok, which could be used for example be used to understand sentiment for brands which could be of interest for investors looking at the retail sector. There were all sorts of complexities which I hadn’t considered before, such as the ability to distinguish between music lyrics and spoken text for tagging, which would be important for isolating sentiment. There was also a vendor, Guideline, focused on ad spend data, for a large number of publicly traded companies from across the world.
Matt Yome, Neudata, discussed the new presidency from the context of the industrials sectors. As has been widely reported market interest in defence companies had risen, and we’ve seen defence spending increase in Europe, such as the UK’s recent announcement. Yome noted that there had been an increase in demand for datasets around geopolitical risk data from a macro perspective but also data around procurement and inventories. It could be tricky to track procurement for heavy equipment, depending on the country. There was also the opportunity to track industrial companies using datasets such as satellite imagery and building permits.
Quant data sourcing and strategy
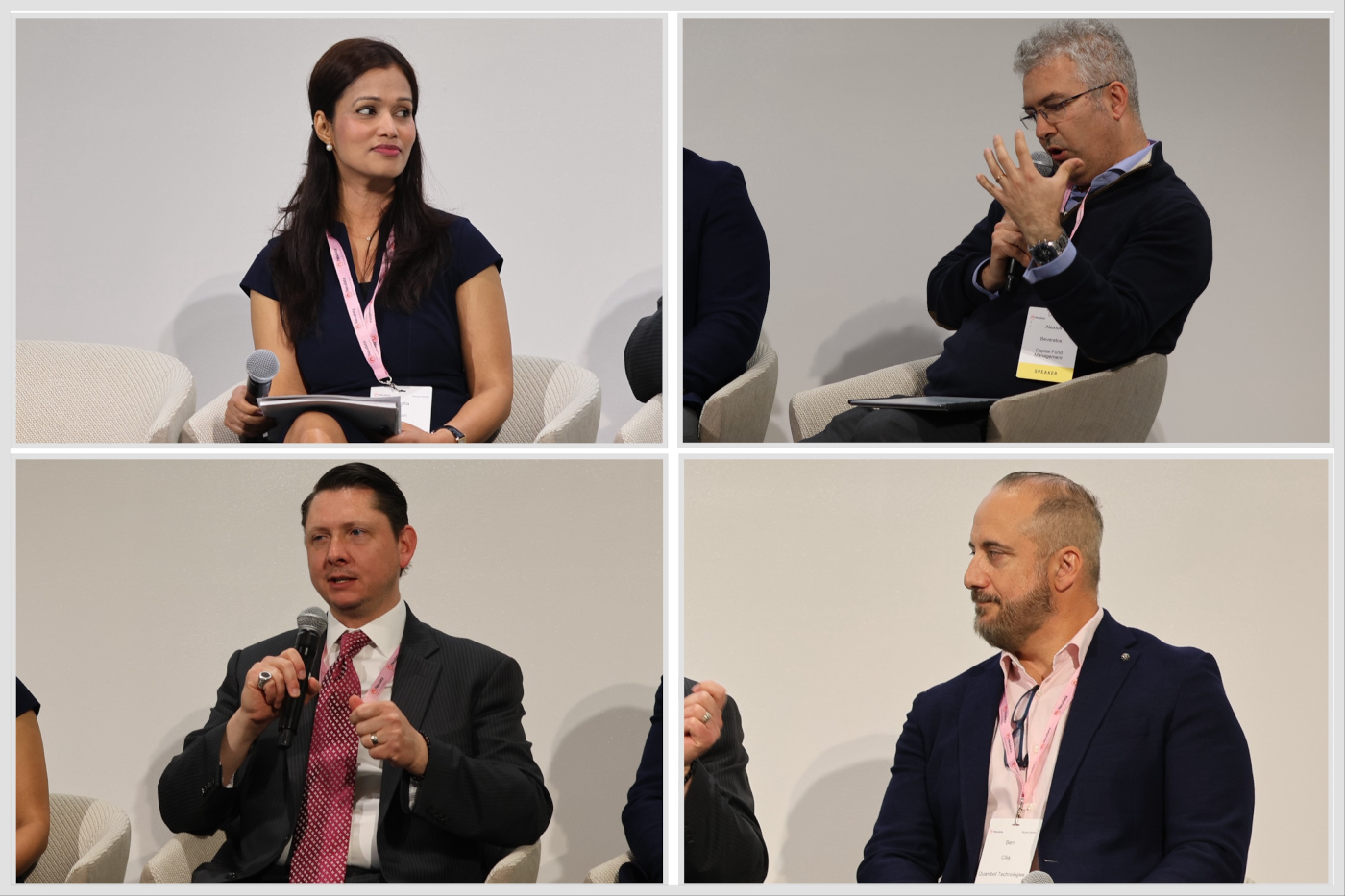
Clockwise – Amrita Tiwari (New York Life IM), Duncan Robinson (Allspring), Ben Cilia (QuantBot) and Alexios Bevratos (CFM)
There was a panel moderated by Amrita Tiwari (New York Life Investment Management) with guests Duncan Robinson (Allspring), Ben Cilia (QuantBot), and Alexios Bevratos (CFM). The subject was around how quants can source new datasets. One initial question was around whether there was too much data. In a sense, it was noted that data is like oil. It’s difficult to discover, and ultimately you are looking for datasets that haven’t yet been sold. It’s better for data vendors to show clients all the possible datasets they have, rather than present what they think the client would be interested in. To find new datasets it was important to stay ahead of new launches to meet people in the industry and so on. Whilst it might be difficult to precisely pinpoint a dataset which would be a market focus (and work tomorrow!) you wanted to have enough datasets to “catch” it when it mattered. One point I’d like to add is that’s a bit like diversification within a portfolio of assets.
There was also the question of crowding and signal decay, which came up in the panel. You could try to allievate such issues with trying something different with the same dataset. If the signal degrades, try to research new signals. When it came to models, one of the panellists noted that they preferred a simple model with great data (rather than vice-versa). Certainly in our use case (inflation forecasting at Turnleaf Analytics), we’d probably agree with this too. Great data is pretty much a prerequisite. Having a more complex data hungry model is not going to help if you don’t have enough data.
There was also a discussion around trials. Even if a trial was “free” for a fund it is not free, because quants need to spend time on it. Whitepapers could help in the evaluation process. Indeed at Turnleaf Analytics, we have found that providing use cases has helped clients more easily evaluate our dataset. It’s not that clients will necessarily run precisely the same use cases/trading strategies that we have found for our data, but it’s more to provide a nice example of how to use our forecast data. It was also important for data vendors to timestamp the data and note for example any specific lags in their data. More breadth and history was always nice too.
Conclusion
I very much enjoyed going to Neudata New York. In particular, many of the discussions I had at the event, ended up being quite different to those I had in Neudata London. The agenda was different, and even if one or two of the topics were the same, having different moderators and panellists resulted in very different discussions.
In particular, it was great to hear the interview of Abraham Thomas, by Mark Fleming-Williams. Whilst the alt data industry is still relatively “young” compared to more traditional financial datasets, it was interesting to hear Abraham’s perspective after his involvement in the industry from its earlier days. Let’s see how the alt data (and data!) industry in finance changes over the coming year.