I recently attended the Neudata conference on alternative data in London. I had last gone quite a few years ago, and I was pleasantly surprised about how much bigger the event had become. Alternative data has become an important part of the financial services industry. As we’ve seen in recent days, with the various tariff announcements, having a quick understanding of how the economy is getting impacted is crucial. Indeed, at Turnleaf Analytics we’ve had many client requests recently around our inflation forecasts. We’ve recently increased the frequency of our inflation forecasts to weekly to cater to this upsurge in interest in timely forecasts around the economy. In this piece, I’ll try to give a summary of the various discussions at the conference. Whilst my write up is inevitably incomplete, and there is a large amount of summarisation, I hope it at least gives a flavour of the event. I’ve tried to pick out the most important points in my view (rather than electing to use ChatGPTs judgement on this!)
The event began with a summary of the state of the alt data market given by Ian Webster from Neudata. He noted that the market could be as large as 12.5bn USD a year, although the lower bound in their estimate could be around 2.5bn USD. He noted that alt data was a growing sector, and spending growth in the area was 33%. Newer funds tended to buy more data, and it was possible that quant funds might test data multiple times before buying. Vendors are also bullish on the alt data market. Perhaps unsurprisingly, the breakout datasets included those around text/LLMs, as well as digital ad intelligence, and alternative CPI forecasts (which tallies with the type of the interest we’ve been seeing from client in Turnleaf Analytics inflation forecast datasets).
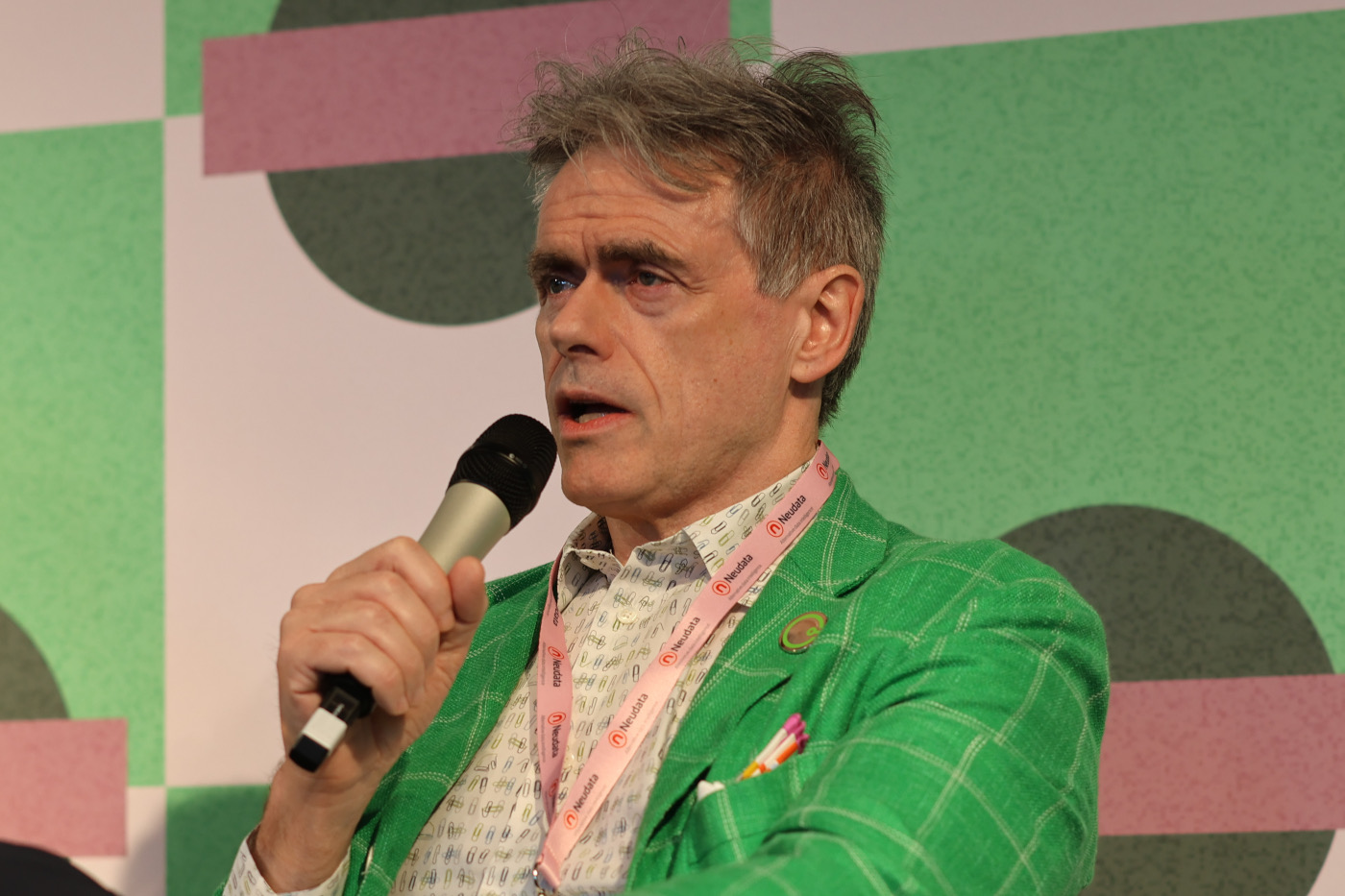
Paul White, QuantBot
Ian continued the conference with Paul White from QuantBot. The title of this session was “Future Proofing: competing in an AI driven constantly changing quant landscape. White went through his career from Morgan Stanley to Merrill Lynch, and then on to founding QuantBot. The tools had changed over time from Perl to these days Python (as well as of course the use of machine learning). Ultimately the P&L of a fund was the sum of people, data and compute. Hence, getting access to high quality data could be an important way to generate P&L. Research is tough, given that the vast majority of it won’t work out of sample. Even if a dataset does have alpha, it might be correlated to existing strategies. Feedback can vary between funds, but ultimately, if a fund buys a dataset it suggests it “works”. Whilst ML and data science was not necessarily new as a tool in quant space, new tools like LLMs were making it easier to do NLP and also helped on the coding side too. Indeed, at Turnleaf Analytics, we’ve found adopting LLMs to help with coding has been incredibly useful. It is possible for multiple teams to use the same data, but end up with uncorrelated strategies, because of the many small details that go into how you look at a dataset.
There was a session with Mark Fleming Williams (CFM), Amy Dafnis (Rokos) and Lee Murison (Jupiter Asset Management) alongside Henry Scherman (Neudata) discussing vendor’s approach to selling to data buyers. There were a lot of interesting points raised in the panel, for example the point that clients were different. Quants will want different things to discretionary funds to asset managers to the various pod shops. Hence, vendors needed to be aware of this when selling data. Even if a dataset was thematic, it might still be useful for a fund to have to bring out when the theme becomes relevant, given that it takes time to onboard a dataset. Point-in-time was of particular relevance from a quant perspective. Then the question of feedback came up after trials. Again it was noted that purchasing a dataset gave a signal, even if details of “alpha” found are of course not shared with the client.
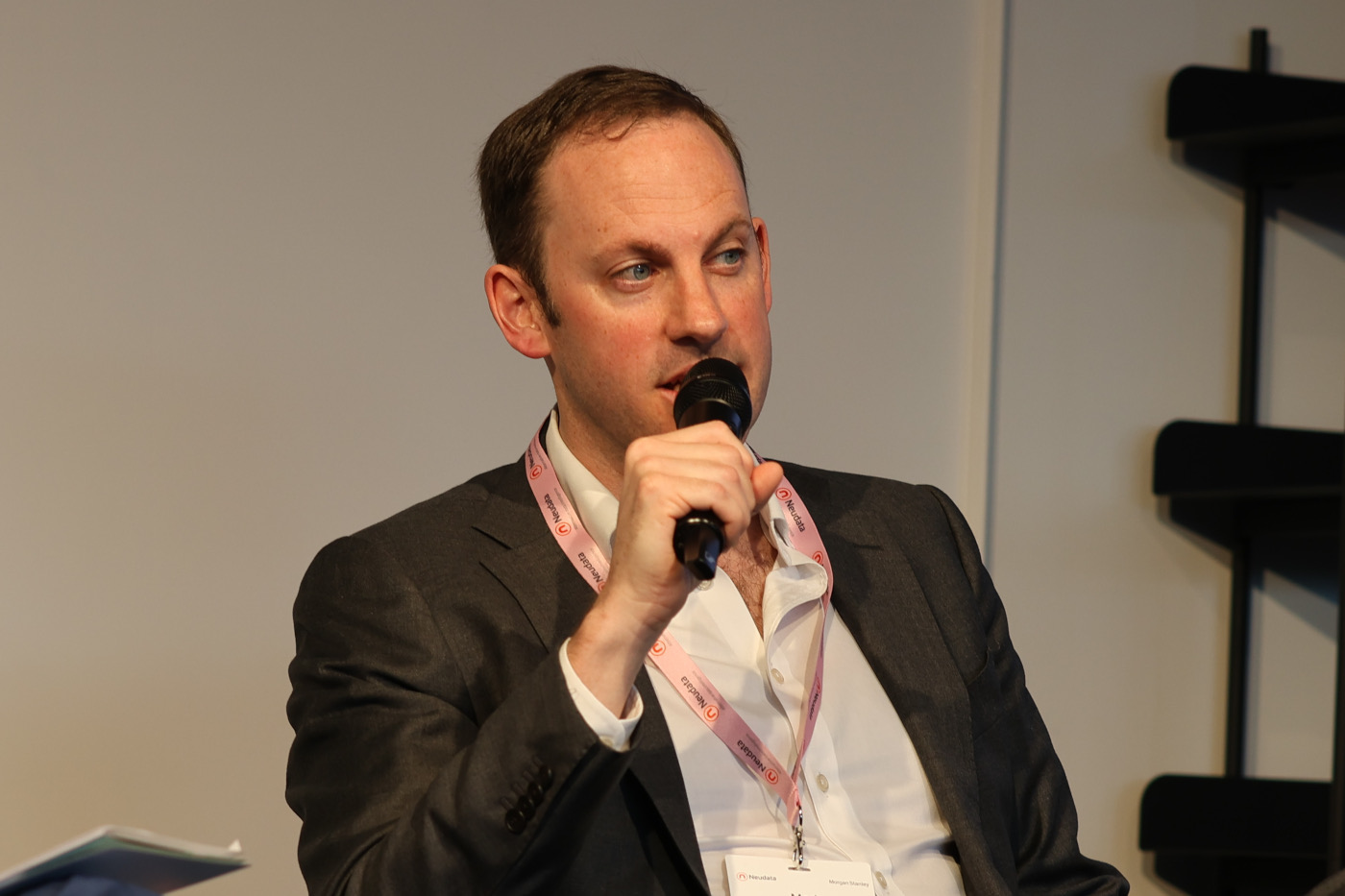
Mark Fleming Williams, CFM
There were a number of Shark Tank sessions showcasing new vendors, the datasets ranged from measuring R&D talent (Zeki Data) to product reviews in the form of Trustpilot. Whilst I’m no expert on equities, I found the Trustpilot presentation particularly interesting. It covers over 300 millions review of products, and for each review there are many different points that can be extracted. They also noted that they had presence in a number of verticals. Finn Cousins (Neudata) did a deep dive on tracking grocery retailers with transaction data. He noted the various forms, which included card data, receipt data and also B2B data from advertising (something which I hadn’t thought of before). He noted how fuel sales were very volatile given the underlying price is volatile, but this was not always stripped out by vendors. Receipt data could be inconsistent between different vendors. Having receipt and panel data (ie. shoppers scanning items) could give specific insights when it came to understanding the mix of discretionary vs. non-discretionary sales at retails. Whilst his session was focused towards tracking grocery data with multiple types of transaction datasets, the points he was making were relevant in many scenarios. Often we need to combine many datasets together to come up with insights, given they augment each other. Indeed, we’ve found this when doing our inflation forecasting. This is a relevant point not purely for alternative data, but also I would add more traditional datasets.
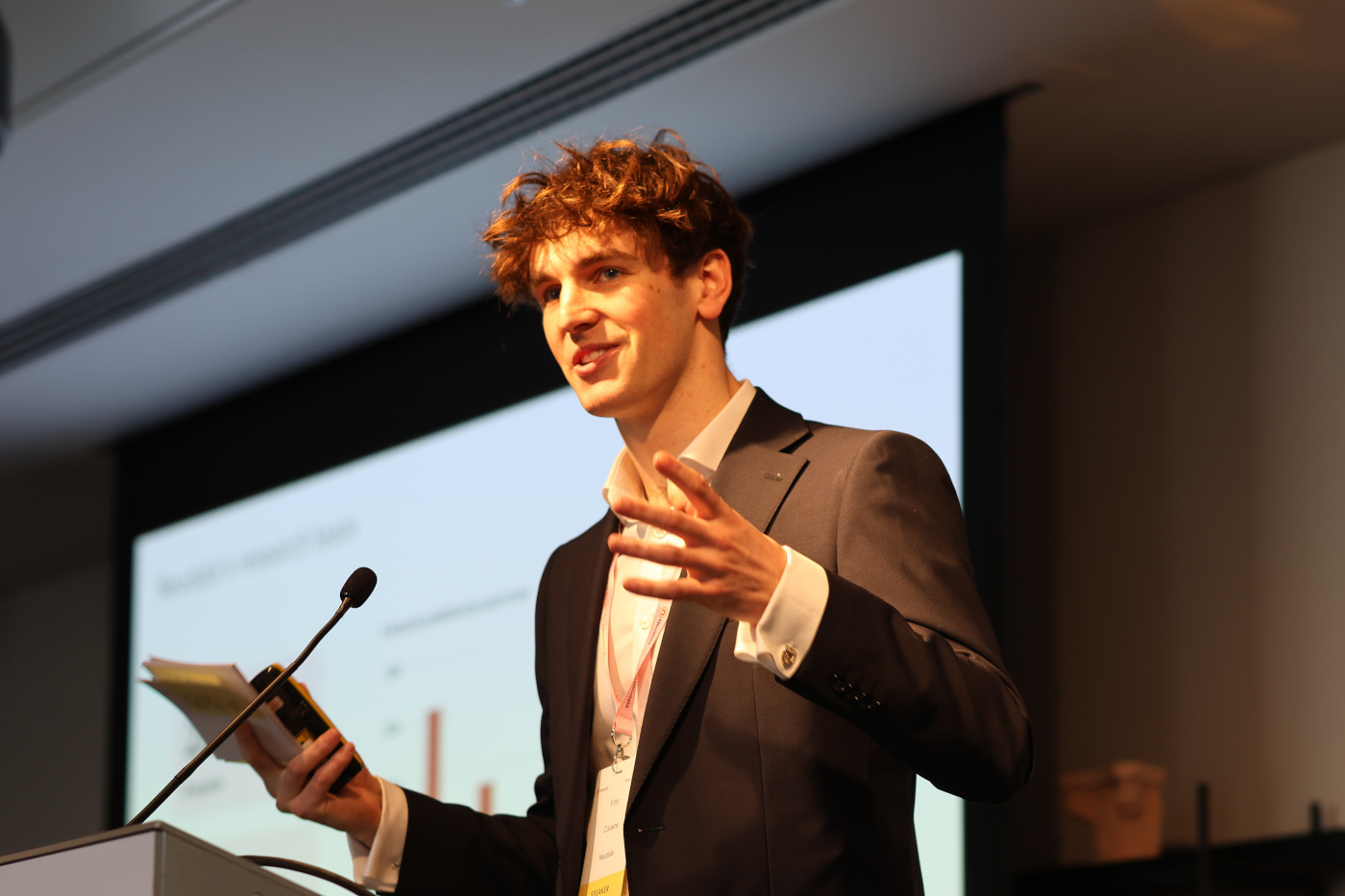
Finn Cousins, Neudata
A later sessions chaired by Sophie Beland (Morgan Stanley), brought together Vik Bansal (Centiva Capital), Florian Koch (Lynx) and Krisina Usaite (Robeco) to discuss the future of data through a quant lens. It was noted how over the years, there has been a difference in what data vendors provided. Whilst in the past it was for data vendors to give anything other than data, they were going up the chain and providing for example signals. Funds are also more open to receiving these additional services. There was of course the perennial question of build vs buy, with a bias to buy, although this was tempered with the point that sometimes the raw data isn’t available. It also depended on the dataset. Whereas in the past sentiment data was often bought, LLMs made it easier to build your own (there is the caveat though that you’d need the underlying text). As mentioned in other sessions, the ability of LLMs to help generate code was useful. The question of crowding and alpha decay came up with datasets. It was noted, consistent with other sessions, that even with the same dataset the correlation did not need to be huge. Crowding was not a big problem, unless a strategy was low capacity. Sometimes crowding could indeed be good, such as with trend following. One point which was repeated across more than one session, was the geography of alt datasets. There could be a lot of coverage for the US, and to a less extent Europe. In EM there was not much. Indeed, that is one of the reasons, why at Turnleaf Analytics, we’ve spent a lot of time expanding our universe of countries for which we forecast inflation to 35 across both EM and DM, extending to countries such as Egypt.
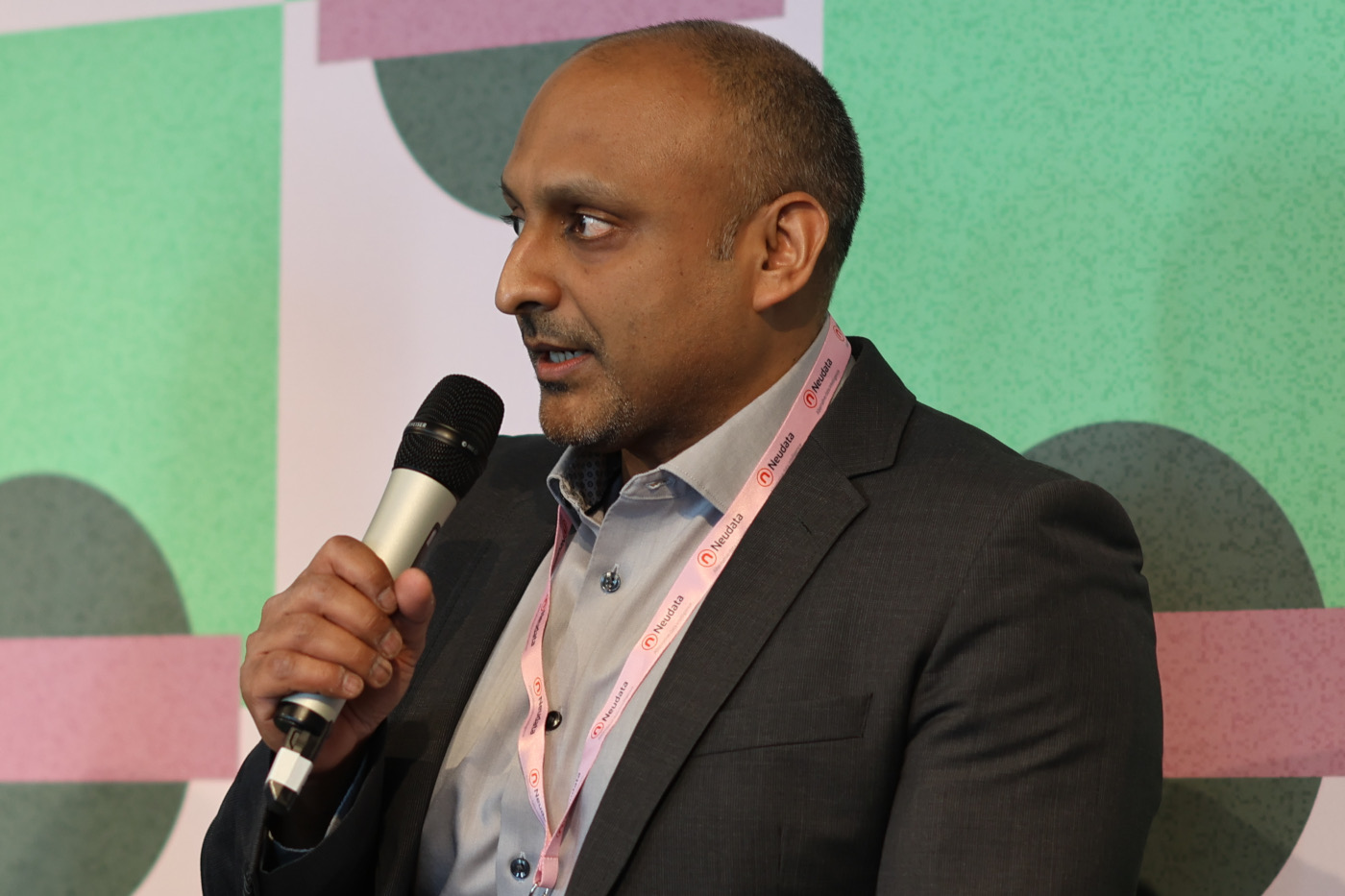
Vik Bansal, Centiva Capital
In conclusion, there seems to be a lot of focus on alt data these days, more so than the past when Alexander Denev and I published The Book of Alternative Data. Also I would say that judging from the attendance at the event, it wasn’t purely quants who seem to be looking at alt data. These days it’s also an area of interest for discretionary investors. It will be interesting to see how the alt data landscape changes over the coming year at the next Neudata event in London. I also suspect that the recent announcements on tariffs, will encourage people to look at alt data more, to get a timely read on the impact of tariffs.